When working long hours isn’t the (only) problem.
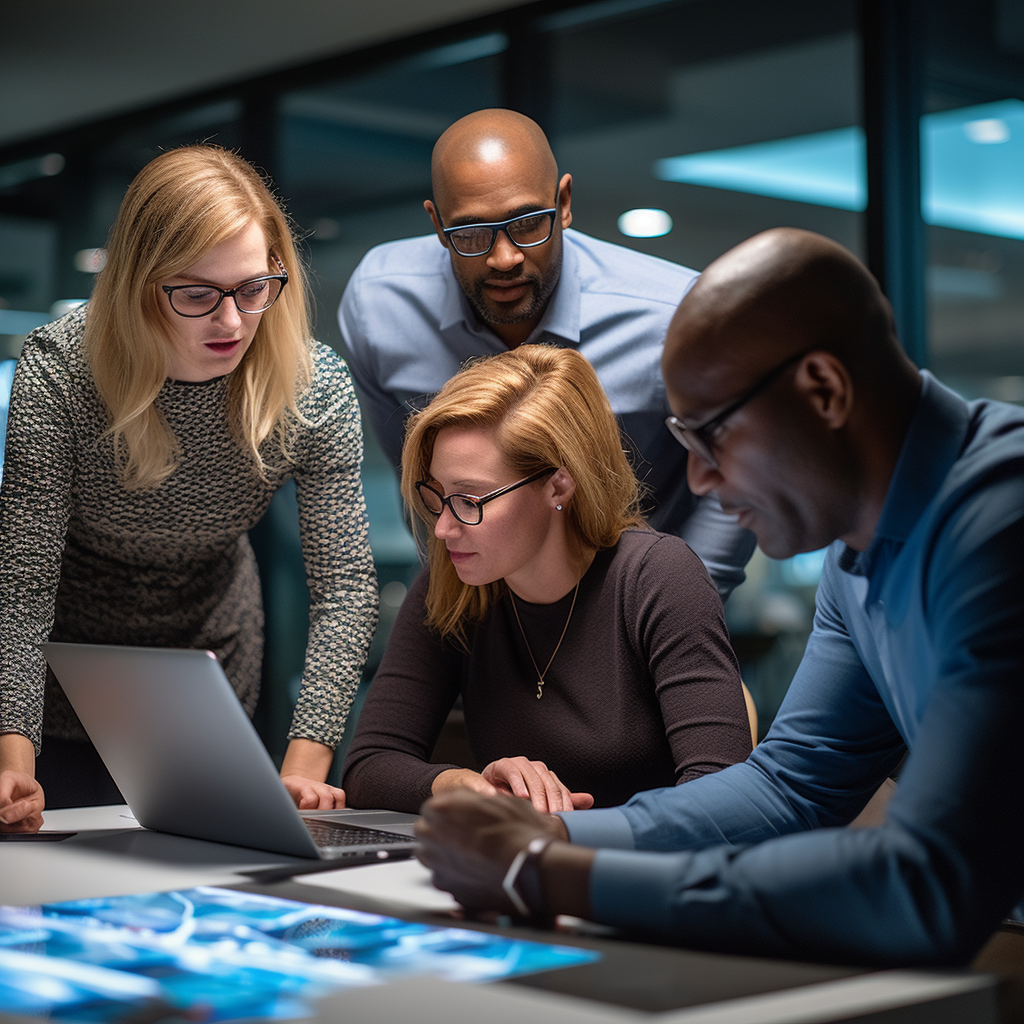
Let’s start with the easy part. We all agree that stress, anxiety, and ultimately burnout are detrimental to the well-being of employees. The correlation with workload is well-known: employees who feel overloaded with work are 30% more likely to experience burnout. This burnout costs $125 billion to $190 billion per year in healthcare costs.
If the goal is to prevent burnout, then how do we find where it’s happening?
There are various ways to assess burnout trends within organizations using research tools such as Maslach Burnout Inventory (MBI). One problem with MBI and employee surveys is that they are confidential and need to remain this way for ethical reasons. Christine Maslach the author of the MBI warned about this in a 2021 Harvard Business School article:
Even more troubling is the misuse of MBI scores to identify (sometimes publicly) people who are “diagnosed” as burned out and who therefore need to be dealt with in some way (“you should seek counseling,” “your team needs to shape up,” “you should quit if you can’t handle the job”)….. the use of an individual’s scores in this way is not acceptable.
The use of employee surveys or pulse research (“How likely are you to get all your work done within the 40-hour work week?”) is also problematic. Putting aside bias and other methodological flaws, surveys are done at a group level and average data is not actionable.
Using data (in the wrong way)
Microsoft Viva does a decent job tracking productivity metrics related to working hours, but the link to burnout is less clear.
That’s because there is a grey area. People work long hours for many reasons and the cause/effect of long hours and burnout is not a given. The salesperson with customers in inconvenient time zones considers long hours a necessity. A web developer may need to block out 3 hours during weekends to catch up on emails and admin work.
Do we use an average of 50, 60, or 70 hours per week to define excessive? Without context, “excessive” is difficult to quantify.
AI in People Analytics
The advantage of using Artificial Intelligence is that it goes beyond simplistic assumptions: longer hours increase the likelihood of burnout. It can, but it doesn’t have to.
Here’s how AI works. Machine Learning algorithms analyze hundreds of variables including meeting requests sent, Slack messages, organizational changes, etc.
The next part is often missed: the algorithms recognize patterns of data behavior for a particular population (company, team, local office, etc.). The next step -and perhaps the magic - is to determine the relevance and weighting of each variable at an individual level.
Trained correctly, the algorithm will recognize that there may be a spike in working hours, but that it’s for all team members and it reverts to a normalized amount. It can also be the regular pattern of the individual that normally decreases after about a week. Alternatively, the algorithm may recognize that within a group of 20 employees, one individual is working consistently longer hours and is not utilizing vacation days. Notice that it’s not the absolute number of hours or utilization rate that helps us find burnout – it’s the abnormalities in patterns and employee behavior that are different from what is expected.
The abnormality can be detected in relation to multiple factors. It can be recognized relative to other people (peers or the entire organization) or the environment (holidays, time off, etc.). It can also refer to the employee themselves (relative to past/other behavior).

AI can be used in various ways. The common mistake to avoid is asking AI questions without providing a reference. We get the best results when the algorithm can compare as many data points as possible. Applying AI to human behavior is complex, requiring algorithms to make comparisons with multiple variables simultaneously – the employee themselves, their peers, overall company employees, and other comparisons to the macro-environment.
Related posts
More insights from Watercooler's team of experts.