Rethinking Retention: How Explainable AI Uncovers Group Dynamics
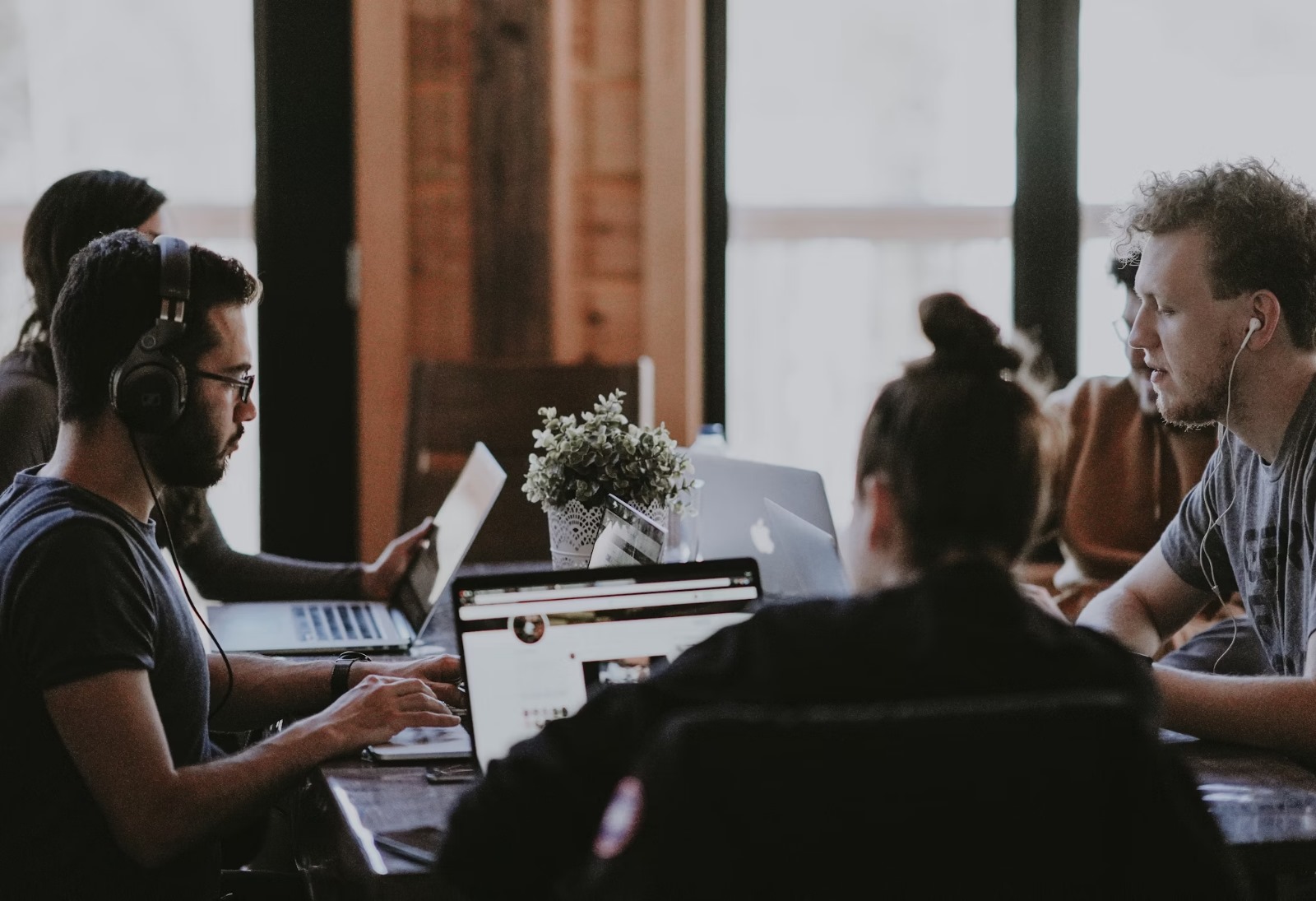
Rethinking Retention: How Explainable AI Uncovers Group Dynamics
It’s often said, “People don’t leave companies, they leave managers.”
While there is truth to this, the relationship between leadership and retention is more complex.
I’ve been thinking a lot about this topic after reading how Nvidia employees are “suffering” from harsh working conditions (long hours, intense pressure, and a lack of work-life balance). But Nvidia’s employees have benefited from stock gains, making many of them millionaires. The result: Nvidia’s retention rate is higher than industry averages.
Is the lesson here that everyone has their price? Or are Nvidia employees the outliers?
Exploring the Bigger Picture:
Nvidia’s case isn’t unique—there are industries like investment banking where people often compete for opportunities to work 80-hour weeks, driven by lucrative salaries and prestige. It’s a reminder that sometimes people stay with managers and companies when they are compensated enough. But does this mean that every organization can expect similar results by simply offering higher pay?
To answer this, we need to dig deeper into how people adapt to their environments.
Adaptive Theory and Group Dynamics
Adaptive Theory provides some insight. It explains how individuals adjust their expectations over time. What may initially seem unbearable—long hours, high pressure, or a stressful workplace—can, over time, become the new normal. As people adapt, their tolerance for difficulty increases, and they recalibrate their perceptions of what’s acceptable. In workplaces like Nvidia, employees may initially feel overwhelmed but eventually normalize these conditions, given the financial incentives.
But beyond individual adaptation, groups also tend to become similar in behavior over time. Within organizations, teams develop their own dynamics, influenced by leadership, workload, and peer interactions. This shared experience reinforces group behavior, whether it's positive or negative.
Explainable AI in People Analytics
This is where Explainable AI comes in. It applies Machine Learning to vast amounts of data from HRIS, Communication, Talent Management Systems, and other internal data sources, using advanced algorithms to uncover patterns and trends in employee behavior. The output provides leaders with HR analytics to understand the most effective ways to engage with their workforce by providing insights drawn from real data. Explainable AI identifies and analyzes the behavioral patterns across different teams and shows how groups behave collectively, what drives their engagement, and where leaders should intervene to improve satisfaction and retention. For example, some teams may thrive with more autonomy and flexibility, while others might need clearer guidance and support.
Conclusion
Nvidia’s case is an interesting example of group dynamics, but not a one-size-fits-all model. While financial incentives alone may drive some employees to work in what may be considered harsh or abusive, this approach is not sustainable for most people.
Most people seek more than just financial rewards; they desire meaningful work, a supportive environment, and a sense of belonging. Explainable AI provides the tools to understand these dynamics,helping leaders create workplaces where engagement and retention can flourish across all teams, not just in exceptional cases.
Related posts
More insights from Watercooler's team of experts.