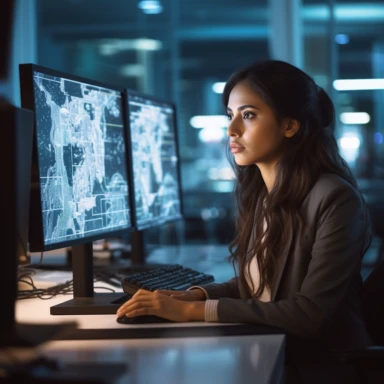
The concept of employee engagement can be traced back to William A. Kahn’s Psychological Conditions of Personal Engagement and Disengagement at Work, published in 1990. [1] Kahn introduced the concept of personal engagement and disengagement at work. In his findings, Kahn stated that people at work “experienced physiological meaningfulness when their task performances included rewarding interpersonal interactions.”
The direct correlation between employee engagement and retention was made in a 2002 study by the Corporate Leadership Council (CLC). The CLC (now part of Gartner) found that highly engaged employees were 87% less likely to leave their organizations than their disengaged counterparts. It is notable that CLC differentiates between two types of engagement. The first is based on rational commitment and is aligned with an employee’s self-interest (financial, developmental, or professional) whereas emotional commitment is based on how employees experience work and the extent to which they believe in what they do.
Multiple academic studies have linked employee engagement with retention, productivity, and business performance. Talent management practices are based on the premise that engaged employees are personally aligned with the goals of the organization and this loyalty is a predictor of positive outcomes.
Much of the evidence is based on analysis of quantitative studies analyzing Employee Net Promoter Scores (eNPS) based on lagging survey data that is susceptible to bias, longitudinal studies that track subjects over time, and qualitative studies such as focus groups and exit interviews which is limited to samples comprised of former employees.
Although the overall correlation between engagement and retention has been established, the methods used are obsolete and there is very little empirical evidence to (1) justify the metrics used to evaluate engagement, (2) explain the optimal level of these metrics, and (3) how these metrics vary from team to team and from one company to another.
This article will explore how Artificial Intelligence (AI) can replace generic guidance that working “excessive” hours results in burnout or that managers and employees should interact "regularly.” We will demonstrate how to replace these amorphous terms with granular-level guidance to managers on the optimal level of employee engagement based on AI analysis of the behavior of the actual employees in the team and organization.
People Analytics based on the Digital Footprint
As employees or entire teams engage with a company's Information Technology systems and Communication platforms, (e.g., email, Slack, and project management tools), they generate a distinctive digital footprint.
This digital footprint is defined as our activities on electronic devices (computers, tablets, smartphones) that are logged on IT servers. Data is processed and categorized as follows: Slack messages (quantity, frequency, response time and timing), emails (sent or received), meetings (duration, count of meeting participants) and GitHub (collaboration activities).
In addition to behavioral data, HRIS data is also used in the AI modeling processes to include information about employee demographics, tenure, compensation, time-off utilization and even performance reviews. It also consists of organizational data including organizational charts, peers and adjacent departures.
In the scenario where the analytics goal is to identify potential resignations, an AI algorithm is applied to historical data from the entire company over a two or three-year period. This data consists of both retained and departed employees. The algorithms are then trained on these employees' digital footprints, scrutinizing over 150 distinct variables for each employee.
The algorithm scans these variables for all employees and looks for unique behavioral patterns or shifts akin to those shown by individuals who resigned. It's crucial to note that the algorithm doesn't examine each variable in isolation. For example, the significance of the variable “excessive work" will be based on whether it is occurring throughout the organization or limited to specific teams or individuals.
Predictions are made at the individual level but are communicated at the team level for the above-mentioned privacy-related concerns (with a minimum of 7 members).
The following graph shows an analysis done for different population groups.
Figure 1 – Resignation Risk (by Team)
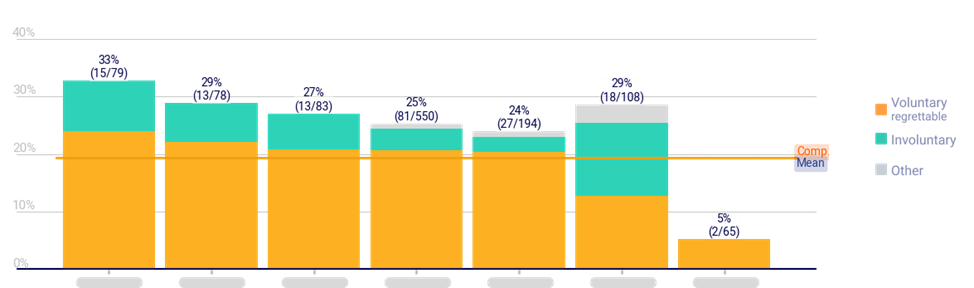
Explainable AI and SHAP Value
It is not only enough to know where resignations are likely to occur (Figure 1) it is the underlying resignation drivers that are of higher importance. For instance, organizations often use exit interviews to identify factors leading to resignation, believing it provides accurate insight into the reasons employees depart. However, this method is inherently flawed as it uses historical data, only captures feedback from a subset of departing individuals and usually not from those who remain, and is susceptible to biased responses from those who may withhold truth to avoid souring relationships with their former employer. It can also lead to the wrong conclusions: instead of focusing on work-life balance and extreme hours, an organization may seek to address managerial and leadership issues, on the assumption that pos can be made from the top down.
When significant investments and decisions are made based on AI, a major concern is that it is a ‘black box’ that cannot be understood. The use of complex models allows us to solve harder challenges with more accuracy, but the complexity of models (depth in most cases) makes it more challenging to explain.
In a linear AI, the output is derived from the sum of the weighted input features. Each feature's contribution is directly determined by its corresponding coefficient or weight. Conversely, in a non-linear model, the features can be interdependent and may have interactions among themselves. This makes the analysis of individual feature contributions more complex.
Explainable Artificial Intelligence (XAI) has emerged as a critical domain within Machiredictionne Learning. It is used to make the decision-making process of models transparent and interpretable. This transparency enables users to comprehend, trust, and effectively manage AI systems.
One of the prominent tools in XAI is SHAP (SHaPley Additive exPlanations)[2] .SHAP values, based on cooperative game theory, provide a unified measure of feature importance by attributing the difference between the prediction and the expected prediction value to each feature in the model. These values ensure a fair allocation of contributions among all features, taking into account potential interactions between them. In other words, it is used to determine how much each player in a cooperative game contributes to the overall success. Each feature (input variable) in the model is considered a ‘player’ and their contribution to the prediction is the ‘payoff’.
For non-linear models, where direct interpretation can be challenging due to featured dependencies, SHAP values have emerged as critical. They offer insights into how specific features influence predictions, even in the presence of intricate interactions.
The following is an example of a resignation prediction for an individual employee with the corresponding SHAP values – i.e., the retention and resignation drivers comprising the overall risk score.
Figure 2 – SHAP Values: (a) Potential Burnout and (b) Failed Onboarding
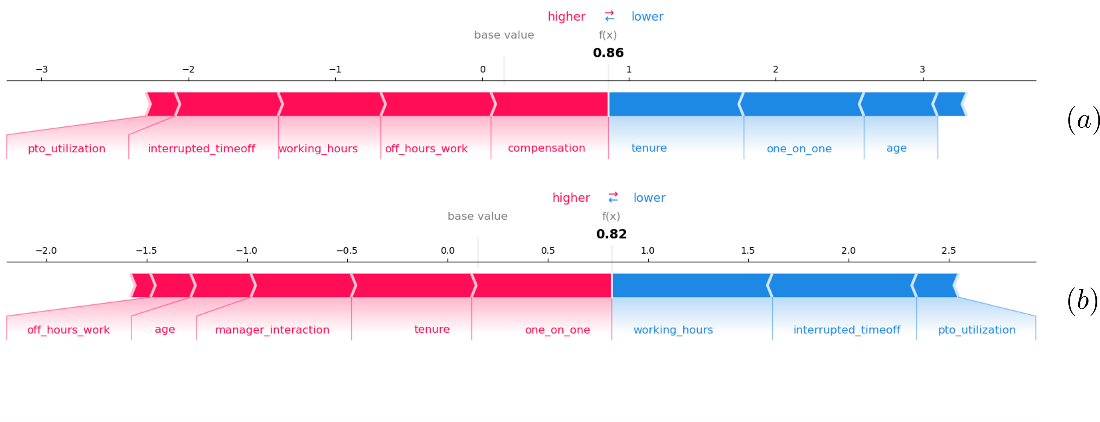
An analysis of the resignation risk itself without an explanation as for the “why”, would make the decisions and derivative actions much more difficult to formulate. Figure 2 above shows two examples of two employees with relatively high resignation risk for completely different reasons. The red sections represent the factors driving resignation for each employee and the blue sections are the mitigating factors likely preventing resignation.
Employee (a) has a high likelihood (0.86)of resignation. The resignation drivers are: (a) relatively low compensation, (b) high incidence of off-hour working, (c) long working hours,and (d) high count of interrupted time-off. The mitigating factors are the employee’s tenure and age as well as sufficient 1on1 with their manager. This pattern reflects potential burnout.
Employee (b) has a high likelihood(0.82) of resignation, but for different reasons. The resignation drivers are: (a) insufficient 1on1’s with their manager (b) infrequent manager interactions, (c) low tenure(they are a recent hire), and (d) age. Mitigating the risk (and lowering the likelihood of resignation) are the employee’s working hours and low incidence of interrupted time off. This pattern reflects potential failed onboarding.
It should also be noted that the model recognizes that the risk is based on the confluence of multiple, independent factors such as age and tenure. It is possible, and even probable, that an employee who is past the onboarding stage or is in a different age group may be more, or less sensitive to one-on-ones and manager interactions.(See Figure 3 below)
Explainable-AI Analysis of Manager Interaction
In the period immediately after COVID-19, many organizations were flexible in allowing work-from-home or hybrid work scenarios. But as uncertainty about the economic environment created concerns about corporate profitability, there was an increase in return to office mandates.
The hybrid or remote work environment is often challenging for managers who may lack the opportunity to engage in person with their teams. Another layer of complexity is that each of the different generations in the workforce requires different management styles. Baby Boomers, Generation X, Millennials, and Generation Z are all characterized by their own work styles and ways of engaging.
According to Gallup’s State of the American Manager Report[3], those employees who are “supervised by highly engaged managers are 59% more likely to be engaged than those supervised by actively disengaged managers.”
But as work models evolve, managers often struggle to find the right balance between communicating regularly and micro-managing. There is no definitive answer to the question “What is the optimal number of interactions between a manager and an employee”.
Explainable-AI can provide a level of specificity that research organizations such as Gallup,that based their research on surveys, are less likely to provide because it analyzes behavioral data at the individual, team, and organizational levels.
The example below shows the relationship between the number of days with employee/manager interactions (per week) and resignation risk. In this example, resignation risk is at its lowest point, when interactions are about ~1 to ~2 times per week and increase when they are below ~1 or exceed ~2.5 times per week (possibly indicating over-communication or even micro-management).
Figure 3 –Analysis of Management Interactions on Flight Risk (Company A)
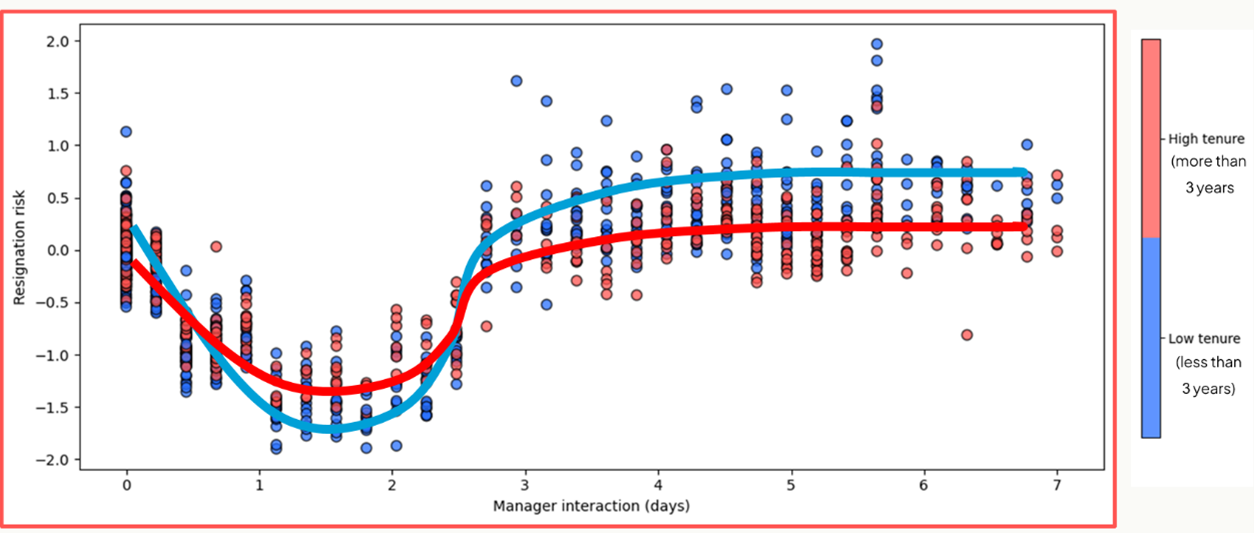
Another important factor is there are differences in the impact of interaction based on the tenure of the employee. Those with higher tenure are less sensitive to management interactions, perhaps reflective of their relative autonomy and experience. Similar studies can be done based on other variables such as age group, geographic location, and job function.
Because AI is based on actual data and not industry trends or survey data, one can expect to see variances between organizations and within organizations. In the example below, which is based on a different company’s data set, a very similar pattern emerges yet with a slight change. In this company, and for the specific team analyzed, the optimal level for communication is between ~2 and ~4 times per week.
Figure 4–Analysis of Management Interactions Contribution to Flight Risk (Company B)

Work Patterns and Resignation Risk
The term Zoom or Meeting Fatigue was popularized during Covid-19. It refers to the exhaustion and stress caused by a high number of meetings, particularly when they are back-to-back or long in duration. This fatigue can result from both physical elements (like sitting in one place for an extended period) and mental elements(like the constant focus required during meetings). It can also lead to burnout and resignation.
There is also a financial cost to meetings. For instance, Shopify has calculated the cost of a typical 30-minute meeting costs the organization between $700 to more than $2,000 depending on attendees’ seniority. [4]
Figure 5–Meetings Time Impact on Flight Risk
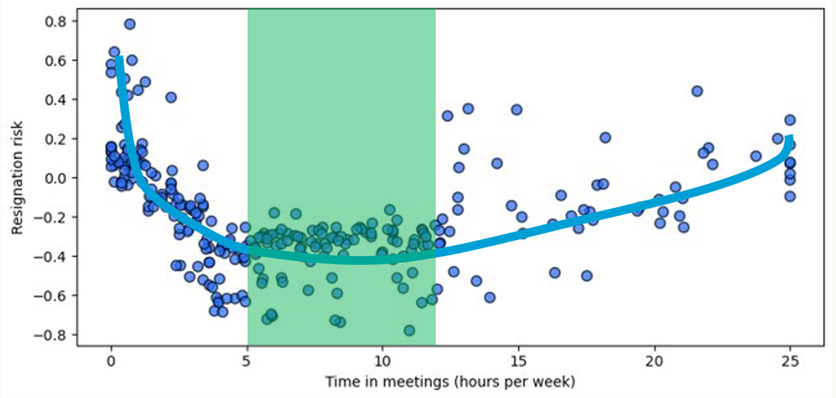
The question of“How many meetings are too many?” is now answerable with Explainable AI. In Figure 5, we see that employee participation in too few meetings is strongly correlated with excessively high resignation levels, which is likely indicative of disengagement or isolation. Similarly, after ~12 hours a week, there is a gradual increase in resignation risk as more hours are spent in meetings.
Managers can utilize this data to optimize the frequency and duration of meetings for each population, to strike a balance that encourages engagement without leading to burnout and resignation. The goal is to identify the so-called sweet spot and avoid extremes on both ends of the spectrum – too few or too many meetings. This approach can be applied to other areas of human behavior, where AI can help us find the optimal point on a U-curve (e.g., engagement levels, span of control network size, etc.).
Multivariate Risk Factor Analysis
Until now, we explored the impact of a single variable on resignation risk.
Human behavior is more complex, requiring an analysis based on a combination of factors.
A basic element of Machine Learning is pattern recognition within multiple variables (from single digits to millions). In Figure 6, we analyze how the combination of multiple factors within one model becomes a strong predictor of resignation. In this case, the algorithm is applied to a combination of both 8+ meeting participants and extreme working hour frequency per week.
Figure 6 – Meetings with 8+ Participants and Extreme Hours

Working excess hours is a well-recognized problem that can impact the health and well-being of employees. A report by the World Health Organization estimated that in 2016, a quarter of a million deaths were attributed to employees working more than 55 hours a week. [5]
Figure 6 shows that a combination of working excessive hours for 4 to 5 days per week and attending meetings with 8+ participants for more than 3.2 hours per week, is associated with elevated resignation risk.
Managers who are provided with this level of information can then take concrete steps to track the working hours of their teams and ensure they are not consistently exceeding a reasonable work week. Similarly, managers should encourage their direct reports to limit the number of attendees per meeting or to split large meetings into smaller, more focused sessions.
Limitations of AI within Behavioral People Analytics
AI is not foolproof and there is always the risk of false positives (when a low-talent risk is incorrectly identified as high talent risk) and false negatives (when the algorithm misses high-risk teams). Furthermore, there are often outside factors that cannot be determined by an AI model. For instance, an employee who has a low risk of resignation could resign based on unexpected health or family circumstances such as the relocation of a spouse.
AI's interpretation of data can sometimes miss out on the nuances of human behavior and relationships. The intricacies of workplace dynamics and individual employee experiences are complex and multi-faceted, and while AI can provide a structured understanding of patterns and correlations, it may not capture the complete picture.
Lastly, there are potential biases in the AI models themselves, which are trained on historical data and could inadvertently perpetuate existing prejudices or unfair practices unless carefully monitored and corrected.
Conclusion
Despite its rapid advances, AI is still in its nascency. The implications for more precise and personalized people management strategies are extensive as the technology continues to mature. In the not-too-distant future, we expect “operating manuals” derived from Behavioral People Analytics that provide people leaders with detailed, prescriptive guidance to help them manage their teams.
While the explanations presented in this article only scratch the surface of AI's potential in managing employee engagement and retention, they offer a glimpse into how technology can bridge the gap between theoretical research and real-world workplace scenarios.
Sources
[1] https://onlinelibrary.wiley.com/doi/10.1002/ail2.61
[2] https://en.wikipedia.org/wiki/Shapley_value
[3] https://www.gallup.com/services/182138/state-american-manager.aspx
[4] https://fortune.com/2023/07/13/shopify-meeting-cost-calculator-expert-warning/
[5] Global, regional, and national burdens of ischemic heart disease and stroke attributable to exposure to long working hours for 194 countries,2000-2016: A systematic analysis from the WHO/ILO Joint Estimates of the Work-related Burden of Disease and Injury. Pega et al. Environment International, (2021)